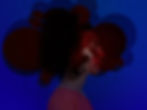
As a Futurist with a background in tech and a former member of Google's groundbreaking team, Spotlight Stories (2016 -2018), I have witnessed firsthand the lack of diversity in the tech industry. During my two years at Google's R&D Lab for Mobile Hardware, ATAP, I realized that I was the only black woman on the team and the first in nearly a decade of the lab's existence.
As a black woman, I understand the importance of representation, especially for those who are underrepresented and feel the pressure to be a positive and reductive pillar of interpersonal interactions in the culture. The lack of diversity in tech is no longer a secret, with the industry mostly employing white and Asian men. This lack of diversity has uncovered major flaws in the technology we use on a daily basis.
For example, Google's Image Recognition and Microsoft Live Labs' Twitter bot have biased tendencies against darker-skinned people, which means governments and companies continue to fail larger and larger groups of people, passing on the racism baton to AI. AI is a powerful tool that can determine credit scores, put people in jail, and even predict the future. However, there have already been instances of AI being racist, such as in biased predictive policing used by police departments, autonomous vehicles not recognizing darker-skinned people as people at all, and AI facial recognition often failing to properly identify people with darker skin tones.
AI is exceptional at reproducing what already exists, but when it comes to creating lasting change, AI lacks the ability to create unprecedented solutions to the new obstacles of our time. Many are concerned about the negative impacts AI will have on society, especially as it relates to issues like diversity and inclusion. There are new voices working in artificial intelligence, and they are fighting to change the way AI learns.
One of these voices is Timnit Gebru, a Black female researcher at Google who was involved in studying the ethical and social implications of artificial intelligence. She was asked to retract her latest research paper on large language models, but she refused and instead wrote a six-page response. When the dispute escalated, she sent two emails, one to her superior and another to a listserver for women who worked in Google Brain. The latter email accused the company of silencing marginalized voices and dismissed Google's internal diversity programs. Gebru was subsequently fired, and her departure ignited a controversy that inflamed Google, which had been facing accusations of mistreating women and people of color. Many AI researchers sympathized with Gebru and found her paper unobjectionable. Thousands of Googlers and outside AI experts signed a public letter castigating the company.
Timnit Gebru's experience has shed light on important lessons for how we approach AI. Her work highlighted the need for diversity and inclusion in the AI field, as well as the need to address biases and ethical concerns in AI systems. Gebru's advocacy for transparency and accountability in AI models and data collection practices has brought attention to the potential harm that can result from unregulated and unchecked AI development. Additionally, Gebru's experience with Google demonstrated the importance of corporate responsibility and ethical leadership in the tech industry. Overall, Gebru's story has emphasized the need for a holistic approach to AI development that takes into account not only technical expertise but also social and ethical considerations.
As we continue to build and improve AI technologies, it is crucial to remember the importance of diversity and representation. Just like any other form of technology, AI is only as good as the data it is trained on. If we want AI to be a force for good and to serve all of humanity, we must ensure that it is trained on a diverse range of data.
Teaching AI the beauty and strength of diversity requires intentional effort and investment. This means actively seeking out diverse datasets and taking steps to ensure that they are properly labeled and annotated to prevent bias. It also means engaging with diverse communities and seeking their input and feedback on AI technologies and their applications.
As AI technology continues to advance, it’s crucial that we train it ethically, especially since AI systems can perpetuate biases and discrimination. However, the task of training ethical AI can seem daunting and inaccessible to the average person.
One approach to training ethical AI is to ensure that the dataset used for training is diverse and representative. As an AI researcher and artist, I am committed to promoting diversity and representation in AI. My collection of Black Art made with AI is one way that I am contributing to this effort. Through this collection, I am training the algorithm to associate Black people with strength, intelligence, resilience, and beauty in all of our shades of brown. In the case of the Black Art Collections project, this means including a wide range of images of black people that showcase their beauty, strength, intelligence, and resilience, instead of relying on negative portrayals from the media or the internet’s echoes of old racist ideologies. By incorporating diverse perspectives and experiences, we can avoid perpetuating harmful biases in AI algorithms.
Another approach is to educate people on the ethical implications of AI and how to mitigate them. For example, AI systems can inadvertently perpetuate discrimination if they are not designed with diversity and inclusion in mind. Therefore, it’s important to train people in the importance of diverse and inclusive datasets, as well as the potential consequences of failing to account for these issues.
In addition to education, it’s important to create tools and resources that make training ethical AI more accessible to the average person. This could include user-friendly software that helps people create diverse datasets or resources that guide people through the process of training and testing AI systems.
Finally, it’s essential to prioritize diversity and inclusion in the development of AI technology. This means ensuring that people from diverse backgrounds are involved in the development process and that ethical considerations are integrated into every stage of development. By prioritizing diversity and inclusion, we can create AI systems that are more ethical and better serve the needs of all people.
By providing diverse and representative data, we can create AI that reflects the diversity of the world we live in. This will lead to more accurate and fair AI technologies that can be used to improve people’s lives in meaningful ways. As we move forward, let us remember that diversity is not only a source of beauty and strength but also a necessary ingredient for creating a better world.